Quadratic constrained quadratic programming: Difference between revisions
No edit summary |
|||
(22 intermediate revisions by the same user not shown) | |||
Line 1: | Line 1: | ||
Author: Jialiang Wang (jw2697), Jiaxin Zhang (jz2289), Wenke Du (wd275), Yufan Zhu (yz2899), David Berroa (deb336) (ChemE 6800 Fall 2024) | Author: Jialiang Wang (jw2697), Jiaxin Zhang (jz2289), Wenke Du (wd275), Yufan Zhu (yz2899), David Berroa (deb336) (ChemE 6800 Fall 2024)<!-- The final wiki submission is edited based on the initial plan --> | ||
Stewards: Nathan Preuss, Wei-Han Chen, Tianqi Xiao, Guoqing Hu | Stewards: Nathan Preuss, Wei-Han Chen, Tianqi Xiao, Guoqing Hu | ||
==Introduction== | ==Introduction== | ||
'''Quadratic programming (QP)''' is one of the oldest topics in the field of optimization that researchers have studied in the twentieth century. The basic QP, where the objective function is quadratic and constraints are linear, paved the way for other forms, such as QCQPs, which also have quadratic constraints | '''[[wikipedia:Quadratic_programming|Quadratic programming (QP)]]''' is one of the oldest topics in the field of optimization that researchers have studied in the twentieth century. The basic QP, where the | ||
[[File:Q.png|thumb|[https://nag.com/solving-quadratically-constrained-quadratic-programming-qcqp-problems/ Solving quadratically constrained quadratic programming (QCQP) problems](NAG,2022)]] | |||
objective function is quadratic and constraints are linear, paved the way for other forms, such as QCQPs, which also have quadratic constraints (McCarl,Moskowitz et al,1977). | |||
A '''[[wikipedia:Quadratically_constrained_quadratic_program|Quadratically Constrained Quadratic Program (QCQP)]]''' can be defined as an optimization problem where the objective function and the constraints are quadratic. In particular, the issue involves optimizing (or minimizing) a convex quadratic function of decision variables with quadratic constraints. This class of problems is well suited to finance, engineering, machine learning, and agriculture because it is easier to model the relationship between variables using quadratic functions. | A '''[[wikipedia:Quadratically_constrained_quadratic_program|Quadratically Constrained Quadratic Program (QCQP)]]''' can be defined as an optimization problem where the objective function and the constraints are quadratic. It emerged as [[wikipedia:Mathematical_optimization|optimisation theory]] grew to address more realistic, [https://zhengy09.github.io/ECE285/lectures/L6.pdf complex problems] of non-linear objectives and constraints. In particular, the issue involves optimizing (or minimizing) a [[wikipedia:Convex_function|convex quadratic function]] of decision variables with quadratic constraints. This class of problems is well suited to finance (Zenios,1993), engineering, machine learning, and agriculture because it is easier to model the relationship between variables using quadratic functions. | ||
* The desire to study QCQPs stems from the fact that they can be used to model practical optimization problems that involve stochasticity in risk, resources, production, and decision-making. For example, in agriculture, using QCQPs can be useful in determining the best crop to grow based on the expected profits and the uncertainties of price changes and unfavourable weather conditions (Floudas,1995). | * The desire to study QCQPs stems from the fact that they can be used to model practical optimization problems that involve stochasticity in risk, resources, production, and decision-making. For example, in agriculture, using QCQPs can be useful in determining the best crop to grow based on the expected profits and the uncertainties of price changes and unfavourable weather conditions (Floudas,1995). | ||
* In finance, the QCQPs are applied in the portfolio's construction to maximize the portfolio's expected returns and the covariance between the assets. It is crucial to comprehend QCQPs and the ways to solve them, such as '''[[wikipedia:Karush–Kuhn–Tucker_conditions|KKT (Karush-Kuhn Tucker)]]''' conditions and '''[[wikipedia:Software-defined_perimeter|SDP (Semidefinite Programming)]]''' relaxations, to solve problems that linear models cannot effectively solve (Bao & Sahinidis,2011). | * In finance, the QCQPs are applied in the portfolio's construction to maximize the portfolio's expected returns and the covariance between the assets. It is crucial to comprehend QCQPs and the ways to solve them, such as '''[[wikipedia:Karush–Kuhn–Tucker_conditions|KKT (Karush-Kuhn Tucker)]]''' conditions and '''[[wikipedia:Software-defined_perimeter|SDP (Semidefinite Programming)]]''' relaxations, to solve problems that linear models cannot effectively solve (Bao & Sahinidis,2011). | ||
In general, analyzing QCQPs is important in order to apply knowledge-based decision-making and enhance the performance and stability of optimization methods in different fields (Zenios,1993). | |||
==Algorithm Discussion== | |||
In mathematical optimization, a quadratically constrained quadratic program (QCQP) is a problem where both the objective function and the constraints are quadratic functions. A related but simpler case is the quadratic program (QP), where the objective function is a convex quadratic function, and the constraints are linear. | |||
=== QP(Quadratic Programming) === | |||
A '''QP''' can be expressed in the standard form: | |||
<math>\begin{matrix}\text{min} & \left ( \frac{1}{2} \right ) x^TPx+q^Tx+r \\ | |||
\text{s.t}. & Gx \preceq h \\ | |||
& Ax=b, | |||
\end{matrix}</math> | |||
where <math>P\in \mathbf{S}_+^n</math> (the set of symmetric positive semidefinite matrices), <math>G\in \mathbb{R}^{m\times n}</math>, and <math>A\in \mathbb{R}^{p\times n}</math>. In this case, we minimize a convex quadratic function over a polyhedron. | |||
=== QCQP(Quadratically Constrained Quadratic Program) === | |||
When the inequality constraints are also quadratic, the problem becomes a '''QCQP.''' This extends the QP formulation to: | |||
<math>\begin{matrix}\text{min} & \left ( \frac{1}{2} \right ) x^TP_0x+q^T_0x+r_0 \\ | |||
\text{s.t}. & \left ( \frac{1}{2} \right ) x^TP_ix+q^T_ix+r_i \leq 0, i=1,\cdots, m \\ | |||
& Ax=b, | |||
\end{matrix}</math> | |||
where <math>P\in \mathbf{S}_+^n</math> for <math>i=0,\cdots,m</math>. A QCQP minimizes a convex quadratic function over a feasible region that is defined by the intersection of ellipsoids when <math>P_I\succ 0</math>. | |||
Notably, quadratic programs are a special case of QCQP, where <math>P_I=0</math> for <math>i=1,\cdots,m</math>, resulting in affine constraints. Similarly, linear programs (LPs) are a subset of QPs, where <math>P=0</math> in the objective function. | |||
There are two primary relaxation techniques for solving QCQPs: | |||
# '''Semidefinite Programming (SDP):''' SDP relaxations replace quadratic constraints with semidefinite constraints, providing a convex approximation of the problem. SDP relaxations are particularly effective for certain QCQP classes, such as those with non-positive off-diagonal elements in the data matrices, where they yield exact solutions. | |||
# '''Karush-Kuhn-Tucker(KKT):''' It builds upon the method of Lagrange multipliers by introducing necessary conditions for optimality that incorporate primal and dual variables. | |||
{| class="wikitable" | {| class="wikitable" | ||
|+ | |+ | ||
Line 16: | Line 51: | ||
|- | |- | ||
|'''KKT(Karush-Kuhn-Tucker)''' | |'''KKT(Karush-Kuhn-Tucker)''' | ||
|KKT is a mathematical optimization method used to solve constrained optimization problems | |KKT is a mathematical optimization method used to solve constrained optimization problems(Ghojogh, Karray et al,2021). | ||
The KKT conditions include stationarity, primal feasibility, dual feasibility, and complementary slackness, making it particularly effective for solving problems with nonlinear constraints. | The KKT conditions include stationarity, primal feasibility, dual feasibility, and complementary slackness, making it particularly effective for solving problems with nonlinear constraints. | ||
|- | |- | ||
|'''SDP (Semidefinite Programming)''' | |'''SDP (Semidefinite Programming)''' | ||
|SDP reformulates a QCQP problem into a semidefinite programming relaxation. | |SDP reformulates a QCQP problem into a semidefinite programming relaxation (Freund,2004).This approach provides a tractable way to solve or approximate solutions to non-convex QCQP problems by “lifting” the problem to a higher-dimensional space and applying SDP relaxation. | ||
It is widely used in areas where global optimization or approximations to non-convex issues are necessary. | It is widely used in areas where global optimization or approximations to non-convex issues are necessary. | ||
|} | |} | ||
[[File:Semidefinite Programming.jpg|thumb|[https://i.ytimg.com/vi/Sm0IUpxBHNs/maxresdefault.jpg Semidefinite Programming]<ref>Cited in the External Link</ref>(Wikipedia,2021)]] | |||
Other relaxations, such as '''Second-Order Cone Programming (SOCP)''', are exact for specific problem classes like QCQPs with zero diagonal elements in the data matrices. These relaxations often produce the same objective value as SDP relaxations(Best,Kale,2000). Moreover, it has been shown that for a class of random QCQPs, SDP relaxations are exact with high probability if the number of constraints grows at a controlled polynomial rate relative to the number of variables. | |||
For non-convex QCQPs, SDP or SOCP relaxations can still provide exact solutions under specific conditions, such as non-positive off-diagonal elements. Additionally, polynomial-time-checkable sufficient conditions exist for SDP relaxations to exactly solve general QCQPs. Randomized QCQPs often exhibit exact semidefinite relaxations as the number of variables and constraints increases proportionally (Bertsima,Darnell et al,1999). | |||
== Numerical Example == | |||
=== Example 1: KKT Approach === | |||
Considering the following numerical example: | |||
== | |||
<math> | <math> | ||
Line 59: | Line 75: | ||
</math> | </math> | ||
Steps: | |||
* Formulate the [[wikipedia:Lagrangian_mechanics|Lagrangian]] and computed the Gradients | |||
* Applied the Stationarity Conditions | |||
* Determined the active constraints using complementary slackness | |||
=== Step 1: | ==== Step 1: Lagrangian Formulation: ==== | ||
The '''Lagrangian formulation''' in optimization is a mathematical framework used to solve constrained optimization problems by incorporating both the objective function and the constraints into a single scalar function, called the '''Lagrangian <math>L</math>''' This formulation introduces '''Lagrange multipliers''' <math>\lambda_i</math>for each constraint, enabling the transformation of a constrained optimization problem into an unconstrained one as follows (Pasandideh, Niaki et al,2015): | |||
<math>L(x, \lambda, \nu)=f_0(x)+\sum_{i=1}^m \lambda_i f_i(x)+\nu^T(A x-b)</math> | |||
where: | |||
<math>f_0(x)</math> is the objective function to be minimized, <math>f_i(x) \leq 0</math> are the inequality constraints | |||
<math>A x=b</math> represents the equality constraints,<math>\quad \lambda_i \geq 0</math> are the Lagrange multipliers associated with the inequality constraints,<math>\quad \nu</math> is the Lagrange multiplier vector for the equality constraints. | |||
Here the example is: | |||
<math> | |||
L(x, \lambda_1, \lambda_2) = (x_1 - 2)^2 + x_2^2 + \lambda_1 (x_1^2 + x_2^2 - 1) + \lambda_2 \left( (x_1 - 1)^2 + x_2^2 - 1 \right). | |||
</math> | |||
For each constraint, | |||
=== | * the complementary slackness is <math> \lambda_i \geq 0, \quad \lambda_i f_i(x)=0, \quad \text { for } i=1,2 </math> | ||
* the primal feasibility is <math> f_i(x) \leq 0 \quad \text { for } i=1,2</math> . | |||
The results for gradient computation are: | |||
* the partial derivatives with respect to <math> x_1</math>: <math> \frac{\partial L}{\partial x_1}=2\left(x_1-2\right)+2 \lambda_1 x_1+2 \lambda_2\left(x_1-1\right)</math> | |||
* the partial derivatives with respect to <math> x_2</math>:<math> | |||
2(x_1 - 2) + 2\lambda_1 x_1 + 2\lambda_2 (x_1 - 1) = | \frac{\partial L}{\partial x_2} = 2x_2 + 2\lambda_1 x_2 + 2\lambda_2 x_2. | ||
</math> | |||
==== Step 2: Stationarity Condition Application: ==== | |||
===== Setting the results to zero ===== | |||
<math> | * <math> | ||
2(x_1 - 2) + 2\lambda_1 x_1 + 2\lambda_2 (x_1 - 1) = 0 | |||
</math> | |||
* <math> | |||
2x_2 + 2\lambda_1 x_2 + 2\lambda_2 x_2 = 0 | |||
</math> | |||
** since <math>x_2 (1 + \lambda_1 + \lambda_2) = 0</math> and <math>\lambda_i \geq 0</math> for <math>i = 1, 2</math>, so <math> | |||
x_2 = 0. | x_2 = 0. | ||
</math> | |||
* with constrains <math> | |||
x_1 \in [0, 1]. | |||
</math> | </math> | ||
===== Substituting ===== | |||
<math>x_2 = 0</math> into the constraints | |||
<math> | <math> | ||
Line 124: | Line 136: | ||
</math> | </math> | ||
===== Problem Solving ===== | |||
<math> | * Substitute <math>x_2 = 0</math> into Equation (1): <math> | ||
x_1 \ | (x_1 - 2) + \lambda_1 x_1 + \lambda_2 (x_1 - 1) = 0. | ||
</math> | |||
** Assume <math>\lambda_1 > 0</math> (since Constraint 1 is active): <math> | |||
x_1^2 - 1 = 0 \quad \Rightarrow \quad x_1 = \pm 1. | |||
</math> | |||
* But from the feasible range, <math>x_1 = 1</math> | |||
** Substitute <math>x_1 = 1</math> into the equation:<math> | |||
\lambda_1 = 1. | |||
</math> | </math> | ||
*** This is acceptable. | |||
* Assume <math>\lambda_2 = 0</math> because Constraint 2 is not active at <math>x_1 = 1</math>. | |||
=== Step | ==== Step 3: Verification ==== | ||
===== Complementary Slackness Verification ===== | |||
* Constraint 1: <math> | |||
\lambda_1 (x_1^2 - 1) = 1 \times (1 - 1) = 0. | |||
</math> | |||
* Constraint 2: <math> | |||
\lambda_2 \left( (x_1 - 1)^2 + x_2^2 - 1 \right) = 0 \times (-1) = 0. | |||
</math> | |||
===== Primal Feasibility Verification ===== | |||
* Constraint 1: <math> | |||
x_1^2 - 1 = 1 - 1 = 0 \leq 0 | |||
</math> | |||
* Constraint 2: <math> | |||
(x_1 - 1)^2 + x_2^2 - 1 = -1 \leq 0. | |||
</math> | |||
==== Step 4: Conclusion ==== | |||
* Optimal Solution: <math> | |||
x_1^* = 1, \quad x_2^* = 0. | |||
</math> | |||
* Minimum Objective Value :<math> | |||
f_0^*(x) = (1 - 2)^2 + 0 = 1. | |||
</math> | |||
''' | === Example 2: SDP- Based QCQP === | ||
'''SDP (Semidefinite Programming)''' here is a convex optimization technique used by relaxing the original problem into a semidefinite form. | |||
The difference: | |||
* For a QCQP problem, the objective is typically: | |||
<math>\operatorname{minimize} f_0(x)=\frac{1}{2} x^T P_0 x+q_0^T x+r_0</math> | |||
== | <math>\text { subject to } f_i(x)=\frac{1}{2} x^T P_i x+q_i^T x+r_i \leq 0, \quad i=1, \ldots, m \text {, }</math> | ||
<math>A x=b </math> | |||
* SDP relaxes the problem by introducing a symmetric matrix $X=x x^T$ and reformulating the problem into the semidefinite cone (where <math>X \succeq 0</math> ensures Xis positive semidefinite): | |||
<math>\operatorname{minimize}\left\langle P_0, X\right\rangle+q_0^T x+r_0,</math> | |||
<math>\text { subject to }\left\langle P_i, X\right\rangle+q_i^T x+r_i \leq 0, \quad i=1, \ldots, m, </math> | |||
<math>X \succeq x x^T, \quad A x=b,</math> | |||
Considering the following numerical example: | |||
<math> \begin{aligned} \text{minimize} \quad & f_0(x) = x_1^2 + x_2^2 \\ \text{subject to} \quad & f_1(x) = x_1^2 + x_2^2 - 2 \leq 0, \\ & f_2(x) = -x_1 x_2 + 1 \leq 0. \end{aligned} </math> | <math> \begin{aligned} \text{minimize} \quad & f_0(x) = x_1^2 + x_2^2 \\ \text{subject to} \quad & f_1(x) = x_1^2 + x_2^2 - 2 \leq 0, \\ & f_2(x) = -x_1 x_2 + 1 \leq 0. \end{aligned} </math> | ||
Interpretation: | Interpretation: | ||
'''Objective:''' | |||
* <math>f_0(x) = x_1^2 + x_2^2</math> is the squared distance from the origin | |||
* A point is found in the feasible region that is as close as possible to the origin. | |||
'''Constraint:''' | |||
* <math>f_1(x) = x_1^2 + x_2^2 - 2 \leq 0</math> restricts <math>(x_1, x_2)</math> to lie inside or on a circle of radius <math>\sqrt{2}</math> | |||
* <math>f_2(x) = -x_1 x_2 + 1 \leq 0 \implies x_1 x_2 \geq 1</math> defines a hyperbolic region | |||
* To satisfy <math>x_1 x_2 \geq 1</math>, both variables must be sufficiently large in magnitude and have the same sign. | |||
'''Calculation Steps:''' | |||
* Lifting and Reformulation | |||
* SDP Relaxation | |||
* Soler Application and Recovering | |||
* Value Optimization | |||
==== Step 1: Stationarity Condition Application: ==== | |||
=== | * Lifted variable introduction:<math> x = \begin{pmatrix} x_1 \\ x_2 \end{pmatrix}, \quad X = x x^T = \begin{pmatrix} x_1^2 & x_1 x_2 \\ x_1 x_2 & x_2^2 \end{pmatrix}. </math> | ||
** If <math>X = x x^T</math>, then <math>X \succeq 0</math> (positive semidefinite) and <math>X</math> is rank-1 | |||
* Objective and Constraints Rewrite in terms of <math>X</math>: | |||
** Objective: <math>x_1^2 + x_2^2 = \langle I, X \rangle</math>, where <math>I</math> is the 2x2 identity matrix. | |||
** Constraint 1: <math>x_1^2 + x_2^2 - 2 \leq 0 \implies \langle I, X \rangle - 2 \leq 0.</math> | |||
** Constraint 2: <math>-x_1 x_2 + 1 \leq 0 \implies X_{12} \geq 1.</math> | |||
The original QCQP is non-convex due to the rank-1 condition on <math>X</math>. Relax the rank constraint and consider only <math>X \succeq 0</math>: | ==== Step 2:SDP Relaxation ==== | ||
The original QCQP is non-convex due to the rank-1 condition on <math>X</math>. | |||
Relax the rank constraint and consider only <math>X \succeq 0</math>: | |||
<math> \begin{aligned} \text{minimize} \quad & \langle I, X \rangle \\ \text{subject to} \quad & \langle I, X \rangle - 2 \leq 0, \\ & X_{12} \geq 1, \\ & X \succeq 0. \end{aligned} </math> | <math> \begin{aligned} \text{minimize} \quad & \langle I, X \rangle \\ \text{subject to} \quad & \langle I, X \rangle - 2 \leq 0, \\ & X_{12} \geq 1, \\ & X \succeq 0. \end{aligned} </math> | ||
==== Step 3: Choose Solver ==== | |||
Solving the SDP, the feasible solution <math>X^*</math> found that achieves the minimum: | Solving the SDP, the feasible solution <math>X^*</math> found that achieves the minimum: | ||
<math> X^* = \begin{pmatrix} 1 & 1 \\ 1 & 1 \end{pmatrix}. </math> | <math> X^* = \begin{pmatrix} 1 & 1 \\ 1 & 1 \end{pmatrix}. </math> | ||
Check that <math>X^*</math> is rank-1: | |||
* Check that <math>X^*</math> is rank-1: | |||
<math> X^* = \begin{pmatrix}1 \\ 1\end{pmatrix} \begin{pmatrix}1 & 1\end{pmatrix} = x^*(x^*)^T, </math> | <math> X^* = \begin{pmatrix}1 \\ 1\end{pmatrix} \begin{pmatrix}1 & 1\end{pmatrix} = x^*(x^*)^T, </math> | ||
* with <math>x^* = (1, 1)</math>. | |||
==== Step 4: Value Optimization ==== | |||
<math> \text{ The orignial QCQP's optimal value is } x^* = (1,1) </math> | |||
* Check feasibility | |||
** <math>x_1^2 + x_2^2 = 1 + 1 = 2 \implies f_1(x^*) = 0 \leq 0.</math> | |||
** <math>x_1 x_2 = 1 \implies f_2(x^*) = -1 + 1 = 0 \leq 0.</math> | |||
** Results“”All constraints are satisfied. | |||
The optimal objective value is: <math>f_0^*(x) = x_1^{*2} + x_2^{*2} = 1 + 1 = 2.</math> | |||
=== Comparasion between Two Examples: === | |||
* '''Accuracy''': Both KKT and SDP methods yielded the exact solution for this convex problem. However, SDP relaxation has the added advantage of handling certain non-convexities under specific conditions, where KKT may fail. | |||
* '''Efficiency''': KKT conditions are computationally faster, making them suitable for real-time applications. In contrast, SDP relaxations are resource-intensive, limiting their use in high-dimensional problems. | |||
* '''Scalability''': The performance of SDP relaxations deteriorates as the problem size increases due to the reliance on matrix computations. | |||
==Application== | |||
=== Application 1: Agriculture (crop selection problem) === | |||
[[File:The quadratic yield response of crops to TRIA in the field.png|thumb|The [https://www.researchgate.net/figure/The-quadratic-yield-response-of-crops-to-TRIA-in-the-field-Potatoes-were-grown-at-Stan_fig1_284807130 quadratic yield response] of crops to TRIA in the field (Ries& Houtz, 1983)]] | |||
In agriculture, quadratic programming plays an essential role in optimizing crop selection and resource allocation. Farmers often face a set of challenging decisions when determining which crops to plant and how to allocate limited resources such as land, water, and labor(McCarl,Moskowitz et el,1977). The decisions become even more complicated when accounting for uncertainties such as fluctuating crop prices, weather conditions, and yield variability. Quadratic programming provides a powerful framework for making these complex decisions more manageable. | |||
==== Objective ==== | |||
In a crop selection problem, [https://www.emerald.com/insight/content/doi/10.1108/03068290210419852/full/html?casa_token=SGYpSyJnQJAAAAAA:upunXwWtsTu1SxBTjLDO7olLjRsF7_GooRy26mSifea9qb33QSreKuYaJfTEQy5aHebBCU5alqRnWVsB5aR8_wkATa3pEsGy0U9q_n5-_pQeSndVNYw the goal is typically to maximize the expected profit while minimizing risks associated with different crops]. | |||
For example, mathematically, the objective could look something like: <math>\operatorname{Maximize} Z=\sum_i p_i x_i-\frac{1}{2} \sum_{i, j} \rho_{i j} x_i x_j</math>. | |||
* Z is the total profit,<math>p_i</math>is the expected profit per unit area of crop i,<math>x_i</math> is the area allocated to crop i,<math>\rho_{i j}</math> is the covariance between the yields or prices of crops. | |||
[https://onlinelibrary.wiley.com/doi/abs/10.2307/1238258?casa_token=4sxkbKbwLBwAAAAA%3ALXWbjj3TzWEtzJUtp0U6CUDI7xkyvfFeUh-YPI5yXB_gw3mWUIIJOvOI8NLHy4ghcqtubgkAZSUTUA Quadratic programming can helps to determine the optimal proportion of each crop to plant], balancing the trade-off between profitability and risk(Pyman,2021). The solution will often suggest a diversified planting strategy that minimizes the overall risk of the farm's production. By including the covariance matrix of crop yields or prices, the quadratic model captures the relationships between crops. For instance, if corn and soybeans tend to have negatively correlated yields due to different water requirements, planting both crops can help mitigate the overall risk. | |||
==== Risk and Contraints ==== | |||
Quadratic programming is particularly effective in modeling these kinds of problems because it can take into account both the expected returns and the covariances between different crops. By using a quadratic objective function, farmers can diversify their crop portfolio to reduce risk—much like how financial portfolio optimization works.This type of analysis allows farmers to make informed decisions that are not solely based on maximizing immediate profits but also on minimizing the potential downside in adverse scenarios (Hossain, Hashim et al,2002). Quadratic programming can also incorporate constraints such as available land, labor availability, crop rotation requirements, and water usage limits, making the solution more practical and aligned with real-world farming conditions. | |||
Another advantage of using quadratic programming in crop selection is its ability to adapt to changing conditions (Pyman, 2021). As new information becomes available—such as updated weather forecasts, market trends, or pest outbreak data—the model can be recalibrated to provide an updated optimal planting strategy. This flexibility is crucial in agriculture, where conditions can change rapidly and have a significant impact on outcomes. | |||
The optimal objective | ===== Risk ===== | ||
The risks can stem from fluctuating market prices, varying yields due to unpredictable weather conditions, and pests or diseases. | |||
* The quadratic term <math>\frac{1}{2} \sum_{i, j} \rho_{i j} x_i x_j</math> represents risk, as it models how different crops interact in terms of their yield or price fluctuations. | |||
* The goal is to maximize the expected returns while minimizing risk by selecting crops with complementary risk profiles. | |||
===== Constraints ===== | |||
A set of constraints is always included that reflect the available resources. For example: | |||
* Land area <math>\sum_i x_i=A</math>, where A is the total available land area | |||
* Water, labor, or other resource limits: These could be modeled as linear constraints based on the crop's resource needs. | |||
* Non-negativity: <math>x_i \geq 0</math> for all crops <math>i_r</math> ensuring that no crop area is negative. | |||
=== Application 2: Production Planning with Diminishing Returns === | |||
[https://www.sciencedirect.com/science/article/pii/S0950705115001392?casa_token=Lh5Tecyr5lcAAAAA:3RM90Wt83hMwaBFbTZRjDeXVHPwicHfp4jpFEvmXeeAQxvO1CXdp6uXu7hYulktZwV5q1kmofw Production planning is another area where Quadratic Programming (QP) has significant applications]. The objective is to maximize profit while considering increasing production costs as output scales up(Deckro & Hebert,2003). By modeling the cost as a quadratic function of production volume, QP provides a realistic way to determine optimal production levels, ensuring that diminishing returns are properly accounted for.In many industries, the production process is characterized by an initial phase of increasing returns, followed by a phase of diminishing returns as production scales up. This can be due to factors such as limited machinery capacity, workforce inefficiencies, or increased costs associated with sourcing additional raw materials(Hall, Heady et al,1968). Quadratic programming helps model these non-linear cost increases by incorporating quadratic terms that reflect the rising marginal costs. This allows decision-makers to find the production level at which profit is maximized without overextending resources or incurring disproportionately high costs. | |||
==== Objective: ==== | |||
The objective is to maximize profit, which is the difference between revenue and costs. A typical quadratic function for the cost might look like this: | |||
<math>\text { Maximize } Z=R(x)-C(x)=p \cdot x-\left(c_0+c_1 \cdot x+c_2 \cdot x^2\right)</math> | |||
* <math>\quad R(x)</math> is the revenue generated from producing x units of output (linear with respect to x ),<math>\quad C(x)</math> is the cost of producing $x$ units (quadratic, reflecting diminishing returns) | |||
* <math>\quad p</math> is the price per unit,<math>c_0</math> is the fixed cost (e.g., rent, utilities), <math>c_1</math>is the linear cost coefficient (e.g., cost of raw materials),<math>c_2</math>is the quadratic cost coefficient (the increasing marginal costs as output increases) | |||
* The term <math>c_2 \cdot x^2</math> models the diminishing returns: as production increases, costs rise faster due to factors like equipment inefficiencies, labor shortages, or higher input costs. | |||
For instance, a manufacturing company may experience economies of scale initially as production increases, leading to lower average costs per unit. However, beyond a certain point, the company might face increased costs due to equipment wear, higher labor requirements, or supply chain constraints. By using a quadratic cost function, QP can identify the optimal production point where the additional cost of producing one more unit starts to outweigh the benefit from additional revenue(Bauer,1970). | |||
==== Constraints: ==== | |||
[[File:Law of Diminishing Returns.png|thumb|[https://utw11041.utweb.utexas.edu/ORMM/supplements/methods/nlpmethod/S2_quadratic.pdf Law of Diminishing Returns(Jensen & Bard,2020)]]]Quadratic programming also allows for the inclusion of various constraints that are common in production planning, such as resource availability, labor hours, and budget limits as follows: | |||
* Resource constraints: The total amount of available resources, such as labor hours, raw materials, or machine capacity:<math>\sum_i r_i \cdot x_i \leq R_{\text {available }}</math> | |||
* Budget limits: Total costs must not exceed a certain budget.<math>\sum_i c_i \cdot x_i \leq B_{\max }</math> | |||
* Production limits: There may be a maximum or minimum limit on the number of units that can be produced, either due to demand or capacity. | |||
This makes the solution more practical and applicable to real-world scenarios. By integrating these constraints, the model ensures that production plans are feasible and align with the company's operational capabilities(Migo-Sumagang, Tan et al,2022). Moreover, QP can be used to simulate different production scenarios, allowing companies to evaluate how changes in costs, resource availability, or market conditions might impact their optimal production strategy. This ability to adapt and reassess production plans in response to changing conditions is crucial for maintaining profitability in dynamic industries. The flexibility of QP enables businesses to stay responsive and make data-driven decisions that optimize both efficiency and profit. | |||
==Conclusion== | ==Conclusion== | ||
In conclusion, Quadratically Constrained Quadratic Programs (QCQPs) are a significant class of optimization problems extending quadratic programming by incorporating quadratic constraints (Bao,Sahinidis,2011). These problems are essential for modeling complex real-world scenarios where both the objective function and the constraints are quadratic. QCQPs are widely applicable in areas such as agriculture, finance, production planning, and machine learning, where they help optimize decisions by balancing competing factors such as profitability, risk, and resource constraints. | In conclusion, Quadratically Constrained Quadratic Programs (QCQPs) are a significant class of optimization problems extending quadratic programming by incorporating quadratic constraints (Bao,Sahinidis,2011). These problems are essential for modeling complex real-world scenarios where both the objective function and the constraints are quadratic. QCQPs are widely applicable in areas such as agriculture, finance, production planning, and machine learning, where they help optimize decisions by balancing competing factors such as profitability, risk, and resource constraints. | ||
The study and solution of QCQPs are critical due to their ability to capture complex relationships and non-linearities, offering a more realistic representation of many practical problems than simpler linear models. Techniques such as | The study and solution of QCQPs are critical due to their ability to capture complex relationships and non-linearities, offering a more realistic representation of many practical problems than simpler linear models. Techniques such as Karush-Kuhn Tucker (KKT) conditions and semidefinite programming (SDP) relaxations provide effective tools for solving QCQPs(Elloumi & Lambert,2019), offering both exact and approximate solutions depending on the problem’s structure. These methods allow for efficient handling of the challenges posed by quadratic constraints and non-linearities. | ||
Looking forward, there are several potential areas for improvement in QCQP algorithms. One direction is the development of more efficient relaxation techniques for solving non-convex QCQPs, especially in large-scale problems where computational efficiency becomes critical. Additionally, there is ongoing research into hybrid methods that combine the strengths of different optimization techniques, such as SDP and machine learning, to improve the robustness and speed of solving QCQPs in dynamic environments. As optimization problems become increasingly complex and data-rich, advancements in QCQP algorithms will continue to play a crucial role in making informed, optimal decisions in diverse applications. | Looking forward, there are several potential areas for improvement in QCQP algorithms. One direction is the development of more efficient relaxation techniques for solving non-convex QCQPs, especially in large-scale problems where computational efficiency becomes critical. Additionally, there is ongoing research into hybrid methods that combine the strengths of different optimization techniques, such as SDP and machine learning, to improve the robustness and speed of solving QCQPs in dynamic environments. As optimization problems become increasingly complex and data-rich, advancements in QCQP algorithms will continue to play a crucial role in making informed, optimal decisions in diverse applications. | ||
==Reference== | ==Reference== | ||
[1] Agarwal, D., Singh, P., & El Sayed, M. A. (2023). The Karush–Kuhn–Tucker (KKT) optimality conditions for fuzzy-valued fractional optimization problems. Mathematics and Computers in Simulation, 205, 861-877, DOI:[https://www.researchgate.net/publication/365290227_The_Karush-Kuhn-Tucker_KKT_optimality_conditions_for_fuzzy-valued_fractional_optimization_problems 10.1016/j.matcom.2022.10.024] | [1] Agarwal, D., Singh, P., & El Sayed, M. A. (2023). ''The Karush–Kuhn–Tucker (KKT) optimality conditions for fuzzy-valued fractional optimization problems''. Mathematics and Computers in Simulation, 205, 861-877, DOI:[https://www.researchgate.net/publication/365290227_The_Karush-Kuhn-Tucker_KKT_optimality_conditions_for_fuzzy-valued_fractional_optimization_problems 10.1016/j.matcom.2022.10.024] | ||
[2] Bao, X., Sahinidis, N. V., & Tawarmalani, M. (2011). [https://link.springer.com/article/10.1007/s10107-011-0462-2 Semidefinite relaxations for quadratically constrained quadratic programming: A review and comparisons] (PDF). ''Mathematical programming'', ''129'', 129-157. | [2] Bao, X., Sahinidis, N. V., & Tawarmalani, M. (2011). [https://link.springer.com/article/10.1007/s10107-011-0462-2 Semidefinite relaxations for quadratically constrained quadratic programming: A review and comparisons] (PDF). ''Mathematical programming'', ''129'', 129-157. | ||
[3] Bose, S., Gayme, D. F., Chandy, K. M., & Low, S. H. (2015). Quadratically constrained quadratic programs on acyclic graphs with application to power flow. IEEE Transactions on Control of Network Systems, 2(3), 278-287,[https://ieeexplore.ieee.org/document/7035094 DOI: 10.1109/TCNS.2015.2401172] | [3] Bauer, L. (1970). A quadratic programming algorithm for deriving efficient farm plans in a risk setting. | ||
[4] Bose, S., Gayme, D. F., Chandy, K. M., & Low, S. H. (2015). ''Quadratically constrained quadratic programs on acyclic graphs with application to power flow''. IEEE Transactions on Control of Network Systems, 2(3), 278-287,[https://ieeexplore.ieee.org/document/7035094 DOI: 10.1109/TCNS.2015.2401172] | |||
[5] Deckro, R. F., & Hebert, J. E. (2003). ''Modeling diminishing returns in project resource planning''. Computers & industrial engineering, ''44''(1), 19-33. | |||
[6] Elloumi, S., & Lambert, A. (2019). ''Global solution of non-convex quadratically constrained quadratic programs''. ''Optimization methods and software'', ''34''(1), 98-114,doi: https://doi.org/10.1080/10556788.2017.1350675 | |||
[ | [7] Freund, R. M. (2004). [https://ocw.mit.edu/courses/15-084j-nonlinear-programming-spring-2004/a632b565602fd2eb3be574c537eea095_lec23_semidef_opt.pdf ''Introduction to semidefinite programming (SDP)''] (PDF). Massachusetts Institute of Technology, 8-11. | ||
[5] Zenios, S. A. (Ed.). (1993). Financial optimization. Cambridge university press,doi:https://doi.org/10.1017/CBO9780511522130 | [8] Ghojogh, B., Ghodsi, A., Karray, F., & Crowley, M. (2021). ''KKT conditions, first-order and second-order optimization, and distributed optimization: tutorial and survey''. arXiv preprint arXiv:2110.01858. | ||
[9] Hall, H. H., Heady, E. O., & Plessner, Y. (1968). [https://onlinelibrary.wiley.com/doi/abs/10.2307/1238258?casa_token=4sxkbKbwLBwAAAAA:LXWbjj3TzWEtzJUtp0U6CUDI7xkyvfFeUh-YPI5yXB_gw3mWUIIJOvOI8NLHy4ghcqtubgkAZSUTUA ''Quadratic programming solution of competitive equilibrium for US agriculture'']. ''American Journal of Agricultural Economics'', ''50''(3), 536-555. | |||
[10] Hossain, S., Hashim Nik Mustapha, N., & Tak Chen, L. (2002). [https://www.emerald.com/insight/content/doi/10.1108/03068290210419852/full/html?casa_token=SGYpSyJnQJAAAAAA:upunXwWtsTu1SxBTjLDO7olLjRsF7_GooRy26mSifea9qb33QSreKuYaJfTEQy5aHebBCU5alqRnWVsB5aR8_wkATa3pEsGy0U9q_n5-_pQeSndVNYw ''A quadratic application in farm planning under uncertainty'']. ''International Journal of Social Economics'', ''29''(4), 282-298. | |||
[11] Jensen, P. A., & Bard, J. F. (2020). ''Nonlinear programming methods. S2 Quadratic programming''. Operations Research Models and Methods. | |||
[12] McCarl, B. A., Moskowitz, H., & Furtan, H. (1977). Quadratic programming applications. ''Omega'', ''5''(1), 43-55. | |||
[13] Migo-Sumagang, M. V., Tan, R. R., Tapia, J. F. D., & Aviso, K. B. (2022). ''Fuzzy mixed-integer linear and quadratic programming models for planning negative emissions technologies portfolios with synergistic interactions''. ''Cleaner Engineering and Technology'', ''9'', 100507. | |||
[14] NAG. (2022). ''Solving quadratically constrained quadratic programming (QCQP) problems''. Retrieved from <nowiki>https://nag.com/solving-quadratically-constrained-quadratic-programming-qcqp-problems/</nowiki> | |||
[15] Pasandideh, S. H. R., Niaki, S. T. A., & Gharaei, A. (2015). [https://www.sciencedirect.com/science/article/pii/S0950705115001392?casa_token=Lh5Tecyr5lcAAAAA:3RM90Wt83hMwaBFbTZRjDeXVHPwicHfp4jpFEvmXeeAQxvO1CXdp6uXu7hYulktZwV5q1kmofw ''Optimization of a multiproduct economic production quantity problem with stochastic constraints using sequential quadratic programming'']. ''Knowledge-Based Systems'', ''84'', 98-107. | |||
[16] Pyman, D. H. (2021). ''The risk-return trade-off to diversified agriculture in Malawi: A quadratic programming approach'' (Doctoral dissertation, Stellenbosch: Stellenbosch University). | |||
[17] Ries, S., & Houtz, R. (1983). ''Triacontanol as a plant growth regulator.'' | |||
[18] Wikipedia. (2021). ''Max paraboloid.svg''. Retrieved from <nowiki>https://en.wikipedia.org/wiki/File:Max_paraboloid.svg</nowiki> | |||
[19] Zenios, S. A. (Ed.). (1993). ''Financial optimization''. Cambridge university press,doi:https://doi.org/10.1017/CBO9780511522130 | |||
==Further Reading== | ==Further Reading== | ||
Line 279: | Line 374: | ||
* [1] Floudas, C. A., & Visweswaran, V. (1995). [https://link.springer.com/chapter/10.1007/978-1-4615-2025-2_5 Quadratic optimization. ''Handbook of global optimization''] ''(PDF)'', 217-269. | * [1] Floudas, C. A., & Visweswaran, V. (1995). [https://link.springer.com/chapter/10.1007/978-1-4615-2025-2_5 Quadratic optimization. ''Handbook of global optimization''] ''(PDF)'', 217-269. | ||
* [2] Xu, H. K. (2003). [https://link.springer.com/article/10.1023/A:1023073621589 An iterative approach to quadratic optimization. ''Journal of Optimization Theory and Applications'']''(PDF)'', ''116'', 659-678. | * [2] [https://link.springer.com/article/10.1007/s10462-022-10273-7 Gunjan, A., & Bhattacharyya, S. (2023). A brief review of portfolio optimization techniques](PDF). ''Artificial Intelligence Review'', ''56''(5), 3847-3886,doi: https://doi.org/10.1007/s10462-022-10273-7 | ||
* [3] Xu, H. K. (2003). [https://link.springer.com/article/10.1023/A:1023073621589 An iterative approach to quadratic optimization. ''Journal of Optimization Theory and Applications'']''(PDF)'', ''116'', 659-678. | |||
In Finance Portfolio Construction: | In Finance Portfolio Construction: | ||
* [1] | * [1] Best, M. J., & Kale, J. K. (2000). [https://www.researchgate.net/publication/238301843_Quadratic_Programming_for_Large-Scale_Portfolio_Optimization Quadratic programming for large-scale portfolio optimization]. In ''Financial Services Information Systems'' (pp. 531-548). Auerbach Publications. | ||
* [2] [https:// | * [2] Bertsimas, D., Darnell, C., & Soucy, R. (1999). [https://www.mit.edu/~dbertsim/papers/Finance/Portfolio%20construction%20through%20mixed%20integer%20programming.pdf Portfolio construction through mixed-integer programming at Grantham](pdf), Mayo, Van Otterloo and Company. ''Interfaces'', ''29''(1), 49-66. | ||
==External Links== | ==External Links== | ||
* [[wikipedia:Convex_function|Convext Quatratic Function]] | |||
* [[wikipedia:Karush–Kuhn–Tucker_conditions|Karush–Kuhn–Tucker conditions]] | |||
* [[wikipedia:Lagrangian_mechanics|Lagrangian mechanics]] | |||
* [https://utw11041.utweb.utexas.edu/ORMM/supplements/methods/nlpmethod/S2_quadratic.pdf Law of Diminishing Rules] | |||
* [[wikipedia:Quadratically_constrained_quadratic_program|Quadratically constrained quadratic program]] | |||
* [https://www.researchgate.net/figure/The-quadratic-yield-response-of-crops-to-TRIA-in-the-field-Potatoes-were-grown-at-Stan_fig1_284807130 Quatratic Programming on Agriculture (crop selection problem)] | |||
* [https://link.springer.com/article/10.1007/s10107-020-01589-9 Semidefinite Programming] | |||
* [https://nag.com/solving-quadratically-constrained-quadratic-programming-qcqp-problems/ Solving quadratically constrained quadratic programming (QCQP) problems] | * [https://nag.com/solving-quadratically-constrained-quadratic-programming-qcqp-problems/ Solving quadratically constrained quadratic programming (QCQP) problems] | ||
* [[wikipedia:Software-defined_perimeter|Software-defined perimeter]] | |||
{| class="wikitable" | {| class="wikitable" | ||
|+ | |+ |
Latest revision as of 03:46, 15 December 2024
Author: Jialiang Wang (jw2697), Jiaxin Zhang (jz2289), Wenke Du (wd275), Yufan Zhu (yz2899), David Berroa (deb336) (ChemE 6800 Fall 2024)
Stewards: Nathan Preuss, Wei-Han Chen, Tianqi Xiao, Guoqing Hu
Introduction
Quadratic programming (QP) is one of the oldest topics in the field of optimization that researchers have studied in the twentieth century. The basic QP, where the
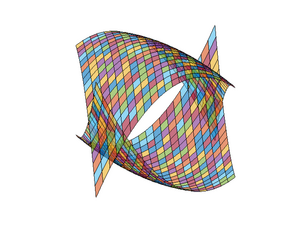
objective function is quadratic and constraints are linear, paved the way for other forms, such as QCQPs, which also have quadratic constraints (McCarl,Moskowitz et al,1977).
A Quadratically Constrained Quadratic Program (QCQP) can be defined as an optimization problem where the objective function and the constraints are quadratic. It emerged as optimisation theory grew to address more realistic, complex problems of non-linear objectives and constraints. In particular, the issue involves optimizing (or minimizing) a convex quadratic function of decision variables with quadratic constraints. This class of problems is well suited to finance (Zenios,1993), engineering, machine learning, and agriculture because it is easier to model the relationship between variables using quadratic functions.
- The desire to study QCQPs stems from the fact that they can be used to model practical optimization problems that involve stochasticity in risk, resources, production, and decision-making. For example, in agriculture, using QCQPs can be useful in determining the best crop to grow based on the expected profits and the uncertainties of price changes and unfavourable weather conditions (Floudas,1995).
- In finance, the QCQPs are applied in the portfolio's construction to maximize the portfolio's expected returns and the covariance between the assets. It is crucial to comprehend QCQPs and the ways to solve them, such as KKT (Karush-Kuhn Tucker) conditions and SDP (Semidefinite Programming) relaxations, to solve problems that linear models cannot effectively solve (Bao & Sahinidis,2011).
In general, analyzing QCQPs is important in order to apply knowledge-based decision-making and enhance the performance and stability of optimization methods in different fields (Zenios,1993).
Algorithm Discussion
In mathematical optimization, a quadratically constrained quadratic program (QCQP) is a problem where both the objective function and the constraints are quadratic functions. A related but simpler case is the quadratic program (QP), where the objective function is a convex quadratic function, and the constraints are linear.
QP(Quadratic Programming)
A QP can be expressed in the standard form:
where (the set of symmetric positive semidefinite matrices), , and . In this case, we minimize a convex quadratic function over a polyhedron.
QCQP(Quadratically Constrained Quadratic Program)
When the inequality constraints are also quadratic, the problem becomes a QCQP. This extends the QP formulation to:
where for . A QCQP minimizes a convex quadratic function over a feasible region that is defined by the intersection of ellipsoids when .
Notably, quadratic programs are a special case of QCQP, where for , resulting in affine constraints. Similarly, linear programs (LPs) are a subset of QPs, where in the objective function.
There are two primary relaxation techniques for solving QCQPs:
- Semidefinite Programming (SDP): SDP relaxations replace quadratic constraints with semidefinite constraints, providing a convex approximation of the problem. SDP relaxations are particularly effective for certain QCQP classes, such as those with non-positive off-diagonal elements in the data matrices, where they yield exact solutions.
- Karush-Kuhn-Tucker(KKT): It builds upon the method of Lagrange multipliers by introducing necessary conditions for optimality that incorporate primal and dual variables.
Name | Brief info |
---|---|
KKT(Karush-Kuhn-Tucker) | KKT is a mathematical optimization method used to solve constrained optimization problems(Ghojogh, Karray et al,2021).
The KKT conditions include stationarity, primal feasibility, dual feasibility, and complementary slackness, making it particularly effective for solving problems with nonlinear constraints. |
SDP (Semidefinite Programming) | SDP reformulates a QCQP problem into a semidefinite programming relaxation (Freund,2004).This approach provides a tractable way to solve or approximate solutions to non-convex QCQP problems by “lifting” the problem to a higher-dimensional space and applying SDP relaxation.
It is widely used in areas where global optimization or approximations to non-convex issues are necessary. |

Other relaxations, such as Second-Order Cone Programming (SOCP), are exact for specific problem classes like QCQPs with zero diagonal elements in the data matrices. These relaxations often produce the same objective value as SDP relaxations(Best,Kale,2000). Moreover, it has been shown that for a class of random QCQPs, SDP relaxations are exact with high probability if the number of constraints grows at a controlled polynomial rate relative to the number of variables.
For non-convex QCQPs, SDP or SOCP relaxations can still provide exact solutions under specific conditions, such as non-positive off-diagonal elements. Additionally, polynomial-time-checkable sufficient conditions exist for SDP relaxations to exactly solve general QCQPs. Randomized QCQPs often exhibit exact semidefinite relaxations as the number of variables and constraints increases proportionally (Bertsima,Darnell et al,1999).
Numerical Example
Example 1: KKT Approach
Considering the following numerical example:
Steps:
- Formulate the Lagrangian and computed the Gradients
- Applied the Stationarity Conditions
- Determined the active constraints using complementary slackness
Step 1: Lagrangian Formulation:
The Lagrangian formulation in optimization is a mathematical framework used to solve constrained optimization problems by incorporating both the objective function and the constraints into a single scalar function, called the Lagrangian This formulation introduces Lagrange multipliers for each constraint, enabling the transformation of a constrained optimization problem into an unconstrained one as follows (Pasandideh, Niaki et al,2015):
where:
is the objective function to be minimized, are the inequality constraints
represents the equality constraints, are the Lagrange multipliers associated with the inequality constraints, is the Lagrange multiplier vector for the equality constraints.
Here the example is:
For each constraint,
- the complementary slackness is
- the primal feasibility is .
The results for gradient computation are:
- the partial derivatives with respect to :
- the partial derivatives with respect to :
Step 2: Stationarity Condition Application:
Setting the results to zero
-
- since and for , so
- with constrains
Substituting
into the constraints
Problem Solving
- Substitute into Equation (1):
- Assume (since Constraint 1 is active):
- But from the feasible range,
- Substitute into the equation:
- This is acceptable.
- Substitute into the equation:
- Assume because Constraint 2 is not active at .
Step 3: Verification
Complementary Slackness Verification
- Constraint 1:
- Constraint 2:
Primal Feasibility Verification
- Constraint 1:
- Constraint 2:
Step 4: Conclusion
- Optimal Solution:
- Minimum Objective Value :
Example 2: SDP- Based QCQP
SDP (Semidefinite Programming) here is a convex optimization technique used by relaxing the original problem into a semidefinite form.
The difference:
- For a QCQP problem, the objective is typically:
- SDP relaxes the problem by introducing a symmetric matrix $X=x x^T$ and reformulating the problem into the semidefinite cone (where ensures Xis positive semidefinite):
Considering the following numerical example:
Interpretation:
Objective:
- is the squared distance from the origin
- A point is found in the feasible region that is as close as possible to the origin.
Constraint:
- restricts to lie inside or on a circle of radius
- defines a hyperbolic region
- To satisfy , both variables must be sufficiently large in magnitude and have the same sign.
Calculation Steps:
- Lifting and Reformulation
- SDP Relaxation
- Soler Application and Recovering
- Value Optimization
Step 1: Stationarity Condition Application:
- Lifted variable introduction:
- If , then (positive semidefinite) and is rank-1
- Objective and Constraints Rewrite in terms of :
- Objective: , where is the 2x2 identity matrix.
- Constraint 1:
- Constraint 2:
Step 2:SDP Relaxation
The original QCQP is non-convex due to the rank-1 condition on .
Relax the rank constraint and consider only :
Step 3: Choose Solver
Solving the SDP, the feasible solution found that achieves the minimum:
- Check that is rank-1:
- with .
Step 4: Value Optimization
- Check feasibility
- Failed to parse (SVG (MathML can be enabled via browser plugin): Invalid response ("Math extension cannot connect to Restbase.") from server "https://wikimedia.org/api/rest_v1/":): {\displaystyle x_1 x_2 = 1 \implies f_2(x^*) = -1 + 1 = 0 \leq 0.}
- Results“”All constraints are satisfied.
The optimal objective value is:
Comparasion between Two Examples:
- Accuracy: Both KKT and SDP methods yielded the exact solution for this convex problem. However, SDP relaxation has the added advantage of handling certain non-convexities under specific conditions, where KKT may fail.
- Efficiency: KKT conditions are computationally faster, making them suitable for real-time applications. In contrast, SDP relaxations are resource-intensive, limiting their use in high-dimensional problems.
- Scalability: The performance of SDP relaxations deteriorates as the problem size increases due to the reliance on matrix computations.
Application
Application 1: Agriculture (crop selection problem)

In agriculture, quadratic programming plays an essential role in optimizing crop selection and resource allocation. Farmers often face a set of challenging decisions when determining which crops to plant and how to allocate limited resources such as land, water, and labor(McCarl,Moskowitz et el,1977). The decisions become even more complicated when accounting for uncertainties such as fluctuating crop prices, weather conditions, and yield variability. Quadratic programming provides a powerful framework for making these complex decisions more manageable.
Objective
In a crop selection problem, the goal is typically to maximize the expected profit while minimizing risks associated with different crops.
For example, mathematically, the objective could look something like: .
- Z is the total profit,is the expected profit per unit area of crop i, is the area allocated to crop i, is the covariance between the yields or prices of crops.
Quadratic programming can helps to determine the optimal proportion of each crop to plant, balancing the trade-off between profitability and risk(Pyman,2021). The solution will often suggest a diversified planting strategy that minimizes the overall risk of the farm's production. By including the covariance matrix of crop yields or prices, the quadratic model captures the relationships between crops. For instance, if corn and soybeans tend to have negatively correlated yields due to different water requirements, planting both crops can help mitigate the overall risk.
Risk and Contraints
Quadratic programming is particularly effective in modeling these kinds of problems because it can take into account both the expected returns and the covariances between different crops. By using a quadratic objective function, farmers can diversify their crop portfolio to reduce risk—much like how financial portfolio optimization works.This type of analysis allows farmers to make informed decisions that are not solely based on maximizing immediate profits but also on minimizing the potential downside in adverse scenarios (Hossain, Hashim et al,2002). Quadratic programming can also incorporate constraints such as available land, labor availability, crop rotation requirements, and water usage limits, making the solution more practical and aligned with real-world farming conditions.
Another advantage of using quadratic programming in crop selection is its ability to adapt to changing conditions (Pyman, 2021). As new information becomes available—such as updated weather forecasts, market trends, or pest outbreak data—the model can be recalibrated to provide an updated optimal planting strategy. This flexibility is crucial in agriculture, where conditions can change rapidly and have a significant impact on outcomes.
Risk
The risks can stem from fluctuating market prices, varying yields due to unpredictable weather conditions, and pests or diseases.
- The quadratic term represents risk, as it models how different crops interact in terms of their yield or price fluctuations.
- The goal is to maximize the expected returns while minimizing risk by selecting crops with complementary risk profiles.
Constraints
A set of constraints is always included that reflect the available resources. For example:
- Land area , where A is the total available land area
- Water, labor, or other resource limits: These could be modeled as linear constraints based on the crop's resource needs.
- Non-negativity: for all crops ensuring that no crop area is negative.
Application 2: Production Planning with Diminishing Returns
Production planning is another area where Quadratic Programming (QP) has significant applications. The objective is to maximize profit while considering increasing production costs as output scales up(Deckro & Hebert,2003). By modeling the cost as a quadratic function of production volume, QP provides a realistic way to determine optimal production levels, ensuring that diminishing returns are properly accounted for.In many industries, the production process is characterized by an initial phase of increasing returns, followed by a phase of diminishing returns as production scales up. This can be due to factors such as limited machinery capacity, workforce inefficiencies, or increased costs associated with sourcing additional raw materials(Hall, Heady et al,1968). Quadratic programming helps model these non-linear cost increases by incorporating quadratic terms that reflect the rising marginal costs. This allows decision-makers to find the production level at which profit is maximized without overextending resources or incurring disproportionately high costs.
Objective:
The objective is to maximize profit, which is the difference between revenue and costs. A typical quadratic function for the cost might look like this:
- is the revenue generated from producing x units of output (linear with respect to x ), is the cost of producing $x$ units (quadratic, reflecting diminishing returns)
- is the price per unit, is the fixed cost (e.g., rent, utilities), is the linear cost coefficient (e.g., cost of raw materials),is the quadratic cost coefficient (the increasing marginal costs as output increases)
- The term models the diminishing returns: as production increases, costs rise faster due to factors like equipment inefficiencies, labor shortages, or higher input costs.
For instance, a manufacturing company may experience economies of scale initially as production increases, leading to lower average costs per unit. However, beyond a certain point, the company might face increased costs due to equipment wear, higher labor requirements, or supply chain constraints. By using a quadratic cost function, QP can identify the optimal production point where the additional cost of producing one more unit starts to outweigh the benefit from additional revenue(Bauer,1970).
Constraints:

Quadratic programming also allows for the inclusion of various constraints that are common in production planning, such as resource availability, labor hours, and budget limits as follows:
- Resource constraints: The total amount of available resources, such as labor hours, raw materials, or machine capacity:
- Budget limits: Total costs must not exceed a certain budget.
- Production limits: There may be a maximum or minimum limit on the number of units that can be produced, either due to demand or capacity.
This makes the solution more practical and applicable to real-world scenarios. By integrating these constraints, the model ensures that production plans are feasible and align with the company's operational capabilities(Migo-Sumagang, Tan et al,2022). Moreover, QP can be used to simulate different production scenarios, allowing companies to evaluate how changes in costs, resource availability, or market conditions might impact their optimal production strategy. This ability to adapt and reassess production plans in response to changing conditions is crucial for maintaining profitability in dynamic industries. The flexibility of QP enables businesses to stay responsive and make data-driven decisions that optimize both efficiency and profit.
Conclusion
In conclusion, Quadratically Constrained Quadratic Programs (QCQPs) are a significant class of optimization problems extending quadratic programming by incorporating quadratic constraints (Bao,Sahinidis,2011). These problems are essential for modeling complex real-world scenarios where both the objective function and the constraints are quadratic. QCQPs are widely applicable in areas such as agriculture, finance, production planning, and machine learning, where they help optimize decisions by balancing competing factors such as profitability, risk, and resource constraints.
The study and solution of QCQPs are critical due to their ability to capture complex relationships and non-linearities, offering a more realistic representation of many practical problems than simpler linear models. Techniques such as Karush-Kuhn Tucker (KKT) conditions and semidefinite programming (SDP) relaxations provide effective tools for solving QCQPs(Elloumi & Lambert,2019), offering both exact and approximate solutions depending on the problem’s structure. These methods allow for efficient handling of the challenges posed by quadratic constraints and non-linearities.
Looking forward, there are several potential areas for improvement in QCQP algorithms. One direction is the development of more efficient relaxation techniques for solving non-convex QCQPs, especially in large-scale problems where computational efficiency becomes critical. Additionally, there is ongoing research into hybrid methods that combine the strengths of different optimization techniques, such as SDP and machine learning, to improve the robustness and speed of solving QCQPs in dynamic environments. As optimization problems become increasingly complex and data-rich, advancements in QCQP algorithms will continue to play a crucial role in making informed, optimal decisions in diverse applications.
Reference
[1] Agarwal, D., Singh, P., & El Sayed, M. A. (2023). The Karush–Kuhn–Tucker (KKT) optimality conditions for fuzzy-valued fractional optimization problems. Mathematics and Computers in Simulation, 205, 861-877, DOI:10.1016/j.matcom.2022.10.024
[2] Bao, X., Sahinidis, N. V., & Tawarmalani, M. (2011). Semidefinite relaxations for quadratically constrained quadratic programming: A review and comparisons (PDF). Mathematical programming, 129, 129-157.
[3] Bauer, L. (1970). A quadratic programming algorithm for deriving efficient farm plans in a risk setting.
[4] Bose, S., Gayme, D. F., Chandy, K. M., & Low, S. H. (2015). Quadratically constrained quadratic programs on acyclic graphs with application to power flow. IEEE Transactions on Control of Network Systems, 2(3), 278-287,DOI: 10.1109/TCNS.2015.2401172
[5] Deckro, R. F., & Hebert, J. E. (2003). Modeling diminishing returns in project resource planning. Computers & industrial engineering, 44(1), 19-33.
[6] Elloumi, S., & Lambert, A. (2019). Global solution of non-convex quadratically constrained quadratic programs. Optimization methods and software, 34(1), 98-114,doi: https://doi.org/10.1080/10556788.2017.1350675
[7] Freund, R. M. (2004). Introduction to semidefinite programming (SDP) (PDF). Massachusetts Institute of Technology, 8-11.
[8] Ghojogh, B., Ghodsi, A., Karray, F., & Crowley, M. (2021). KKT conditions, first-order and second-order optimization, and distributed optimization: tutorial and survey. arXiv preprint arXiv:2110.01858.
[9] Hall, H. H., Heady, E. O., & Plessner, Y. (1968). Quadratic programming solution of competitive equilibrium for US agriculture. American Journal of Agricultural Economics, 50(3), 536-555.
[10] Hossain, S., Hashim Nik Mustapha, N., & Tak Chen, L. (2002). A quadratic application in farm planning under uncertainty. International Journal of Social Economics, 29(4), 282-298.
[11] Jensen, P. A., & Bard, J. F. (2020). Nonlinear programming methods. S2 Quadratic programming. Operations Research Models and Methods.
[12] McCarl, B. A., Moskowitz, H., & Furtan, H. (1977). Quadratic programming applications. Omega, 5(1), 43-55.
[13] Migo-Sumagang, M. V., Tan, R. R., Tapia, J. F. D., & Aviso, K. B. (2022). Fuzzy mixed-integer linear and quadratic programming models for planning negative emissions technologies portfolios with synergistic interactions. Cleaner Engineering and Technology, 9, 100507.
[14] NAG. (2022). Solving quadratically constrained quadratic programming (QCQP) problems. Retrieved from https://nag.com/solving-quadratically-constrained-quadratic-programming-qcqp-problems/
[15] Pasandideh, S. H. R., Niaki, S. T. A., & Gharaei, A. (2015). Optimization of a multiproduct economic production quantity problem with stochastic constraints using sequential quadratic programming. Knowledge-Based Systems, 84, 98-107.
[16] Pyman, D. H. (2021). The risk-return trade-off to diversified agriculture in Malawi: A quadratic programming approach (Doctoral dissertation, Stellenbosch: Stellenbosch University).
[17] Ries, S., & Houtz, R. (1983). Triacontanol as a plant growth regulator.
[18] Wikipedia. (2021). Max paraboloid.svg. Retrieved from https://en.wikipedia.org/wiki/File:Max_paraboloid.svg
[19] Zenios, S. A. (Ed.). (1993). Financial optimization. Cambridge university press,doi:https://doi.org/10.1017/CBO9780511522130
Further Reading
In Algorithm:
- [1] Floudas, C. A., & Visweswaran, V. (1995). Quadratic optimization. Handbook of global optimization (PDF), 217-269.
- [2] Gunjan, A., & Bhattacharyya, S. (2023). A brief review of portfolio optimization techniques(PDF). Artificial Intelligence Review, 56(5), 3847-3886,doi: https://doi.org/10.1007/s10462-022-10273-7
- [3] Xu, H. K. (2003). An iterative approach to quadratic optimization. Journal of Optimization Theory and Applications(PDF), 116, 659-678.
In Finance Portfolio Construction:
- [1] Best, M. J., & Kale, J. K. (2000). Quadratic programming for large-scale portfolio optimization. In Financial Services Information Systems (pp. 531-548). Auerbach Publications.
- [2] Bertsimas, D., Darnell, C., & Soucy, R. (1999). Portfolio construction through mixed-integer programming at Grantham(pdf), Mayo, Van Otterloo and Company. Interfaces, 29(1), 49-66.
External Links
- Convext Quatratic Function
- Karush–Kuhn–Tucker conditions
- Lagrangian mechanics
- Law of Diminishing Rules
- Quadratically constrained quadratic program
- Quatratic Programming on Agriculture (crop selection problem)
- Semidefinite Programming
- Solving quadratically constrained quadratic programming (QCQP) problems
- Software-defined perimeter
Category: NonLinear Programming (NLP) - Quadratic programming |
---|
- ↑ Cited in the External Link