Sparse Reconstruction with Compressed Sensing: Difference between revisions
Molokaicat (talk | contribs) |
Molokaicat (talk | contribs) |
||
Line 40: | Line 40: | ||
If the matrix <math>A \in \mathcal{R}^{M \times N}</math> satisfies the RIP condition of order <math>2k</math> and the constant <math>\delta_{2k} \in [0,1)</math>, there are two distinct <math>k</math>-sparse vectors in <math>\Sigma_{2k}</math>. When they are equal, the restricted isometry property holds. If <math>A</math> is a <math>2k</math>-order RIP matrix, it means that no two <math>k</math>-sparse vectors are mapped to the same measurement vector <math>y</math> by <math>A</math>. In other words, when working with sparse vectors, the RIP ensures that the columns of <math>A</math> are nearly orthonormal. Furthermore, <math>A</math> is an approximately norm-preserving function, which means that it preserves its distance when mapping for <math>k</math>-sparse signals for all or more as <math>\delta_k</math> approaches zero. | If the matrix <math>A \in \mathcal{R}^{M \times N}</math> satisfies the RIP condition of order <math>2k</math> and the constant <math>\delta_{2k} \in [0,1)</math>, there are two distinct <math>k</math>-sparse vectors in <math>\Sigma_{2k}</math>. When they are equal, the restricted isometry property holds. If <math>A</math> is a <math>2k</math>-order RIP matrix, it means that no two <math>k</math>-sparse vectors are mapped to the same measurement vector <math>y</math> by <math>A</math>. In other words, when working with sparse vectors, the RIP ensures that the columns of <math>A</math> are nearly orthonormal. Furthermore, <math>A</math> is an approximately norm-preserving function, which means that it preserves its distance when mapping for <math>k</math>-sparse signals for all or more as <math>\delta_k</math> approaches zero. Candes, Romberg, and Tao <ref name = "CRT 2005"> proved that if <math>x</math> is <math>k</math>-sparse, and <math>A</math> satisfies the RIP of order <math>2k</math> with RIP-constant <math>\delta_{2k} < \sqrt(2) - 1</math>, then <math>l_1</math> gives a unique sparse solution. The <math>l_1</math> convex optimization problem is the same as the solution to the <math>l_0</math> program and can solve by the Linear Program <ref name = "Koep" /> <ref = "Caluccia"/>. Hence, the <math>\ell_1</math> reconstruction problem is as followed which can be solved by basis pursuit <ref name = "CRT 2005"/> <ref name = "Donoho"/>. | ||
<math>\hat{x} = \underset{x \in \Sigma_k}{arg min} \|x\|_1 \quad s.t. \quad y = A x</math> | <math>\hat{x} = \underset{x \in \Sigma_k}{arg min} \|x\|_1 \quad s.t. \quad y = A x</math> |
Revision as of 19:44, 20 December 2021
Author: Ngoc Ly (SysEn 5800 Fall 2021)
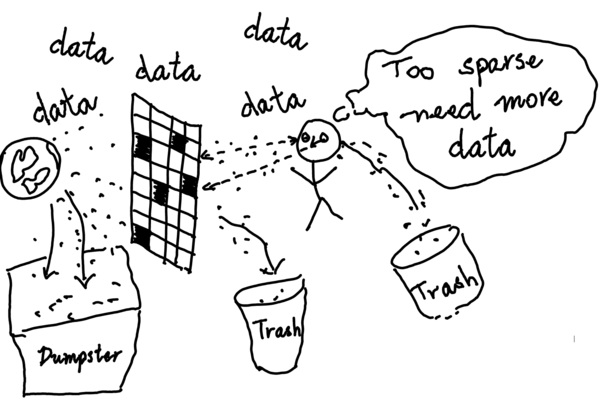
Introduction
sub module goal
The goal of compressed sensing is to solve the underdetermined linear system in which the number of variables is much greater than the number of observations, resulting in an infinite number of signal coefficient vectors for the same set of compressive measurements . The objective is to reconstruct a vector in a given of measurements and a sensing matrix A. Instead of taking a large number of high-resolution measurements and discarding the majority of them, consider taking far fewer random measurements and reconstructing the original with high probability from its sparse representation.
sub modual
Begin with a linear equation , where is a sensing matrix that must be obtained and will result in either exact or approximated optimum solution depending on how it is chosen, is a signal vector with at most -sparse entries, which means has non-zero entries, be an index set, is a compressed measurement vector, , is a noise vector and assumed to be bounded if it exists, and .
sub module sparsity
A vector is said to be -sparse in if it has at most nonzero coefficients. The support of is , and is a -sparse signal when the cardinality . The set of -sparse vectors is denoted by . Consequently, there are different subsets of -sparse vectors. If a random -sparse is drawn uniformly from , its entropy is approximately equivalent to bits are required for compression of [1].
The idea is to search for the sparsest from the measurement vector and a sensing matrix with . If the number of linear measurements is at least twice as its sparsity , i.e., , then there exists at most one signal that satisfies the constraint and produce the correct result for any [2]. Hence, the reconstruction problem can be formulated as an "norm" program.
This optimization problem minimizes the number of nonzero entries of subject to the constraint , that is to find the sparsest element in the affine space [3]. It turns out to be a combinatorial optimization problem, which is NP-Hard because it includes all possible sets of -sparse out of . Furthermore, if noise is present, the recovery is unstable [Buraniuk "compressed sensing"].
Restricted Isometry Property (RIP)
A matrix is said to satisfy the RIP of order if for all has a . A restricted isometry constant (RIC) of is the smallest satisfying this condition [4][5][6].
Under projections through matrix , the restricted isometry property allows -sparse vectors to have unique measurement vectors . If meets RIP, then does not send two distinct -sparse to the same measurement vector , indicating that is a unique solution under RIP.
If the matrix satisfies the RIP condition of order and the constant , there are two distinct -sparse vectors in . When they are equal, the restricted isometry property holds. If is a -order RIP matrix, it means that no two -sparse vectors are mapped to the same measurement vector by . In other words, when working with sparse vectors, the RIP ensures that the columns of are nearly orthonormal. Furthermore, is an approximately norm-preserving function, which means that it preserves its distance when mapping for -sparse signals for all or more as approaches zero. Candes, Romberg, and Tao Cite error: Closing </ref>
missing for <ref>
tag
- ↑ 1.0 1.1 Laska Jason Noah. Rice university regime change: Sampling rate vs. bit-depth in compressive sensing, 2011.
- ↑ 2.0 2.1 Giulio Coluccia, Chiara Ravazzi, and Enrico Magli. Compressed sensing for dis- tributed systems, 2015.
- ↑ 3.0 3.1 Niklas Koep, Arash Behboodi, and Rudolf Mathar. An introduction to compressed sensing, 2019.
- ↑ 4.0 4.1 Emmanuel Candes, Justin Romberg, and Terence Tao. Stable signal recovery from incomplete and inaccurate measurements. March 2005.
- ↑ Cite error: Invalid
<ref>
tag; no text was provided for refs namedCandes Tao
- ↑ 6.0 6.1 Richard Baraniuk, Mark Davenport, Ronald DeVore, and Michael Wakin. A simple proof of the restricted isometry property for random matrices. 28:253–263, 2008.
- ↑ Angshul Majumdar. Compressed sensing for engineers. Devices, circuits, and systems.
- ↑ Simon Foucart and Holger Rauhut. A mathematical introduction to compressive sens- ing. Applied and numerical harmonic analysis. Birkhäuser, New York [u.a.], 2013.
- ↑ D. L. Donoho. Compressed sensing. 52:1289–1306, 2006.
- ↑ E. J. Candes, J. Romberg, and T. Tao. Robust uncertainty principles: exact signal reconstruction from highly incomplete frequency information. 52:489–509, 2006.
- ↑ Mohammed Rostami. Compressed sensing with side information on feasible re- gion, 2013.
- ↑ Richard G. Baraniuk. Compressive sensing [lecture notes]. IEEE Signal Processing Magazine, 24(4):118–121, 2007.
- ↑ Martin Burger, Janic Föcke, Lukas Nickel, Peter Jung, and Sven Augustin. Recon- struction methods in thz single-pixel imaging, 2019.
- ↑ J. K. Pillai, V. M. Patel, R. Chellappa, and N. K. Ratha. Secure and robust iris recognition using random projections and sparse representations. 33:1877–1893, 2011.
- ↑ Y. V. Parkale and S. L. Nalbalwar, “Sensing Matrices in Compressed Sensing.” pp. 113–123, 2020. doi: 10.1007/978-981-32-9515-5_11.